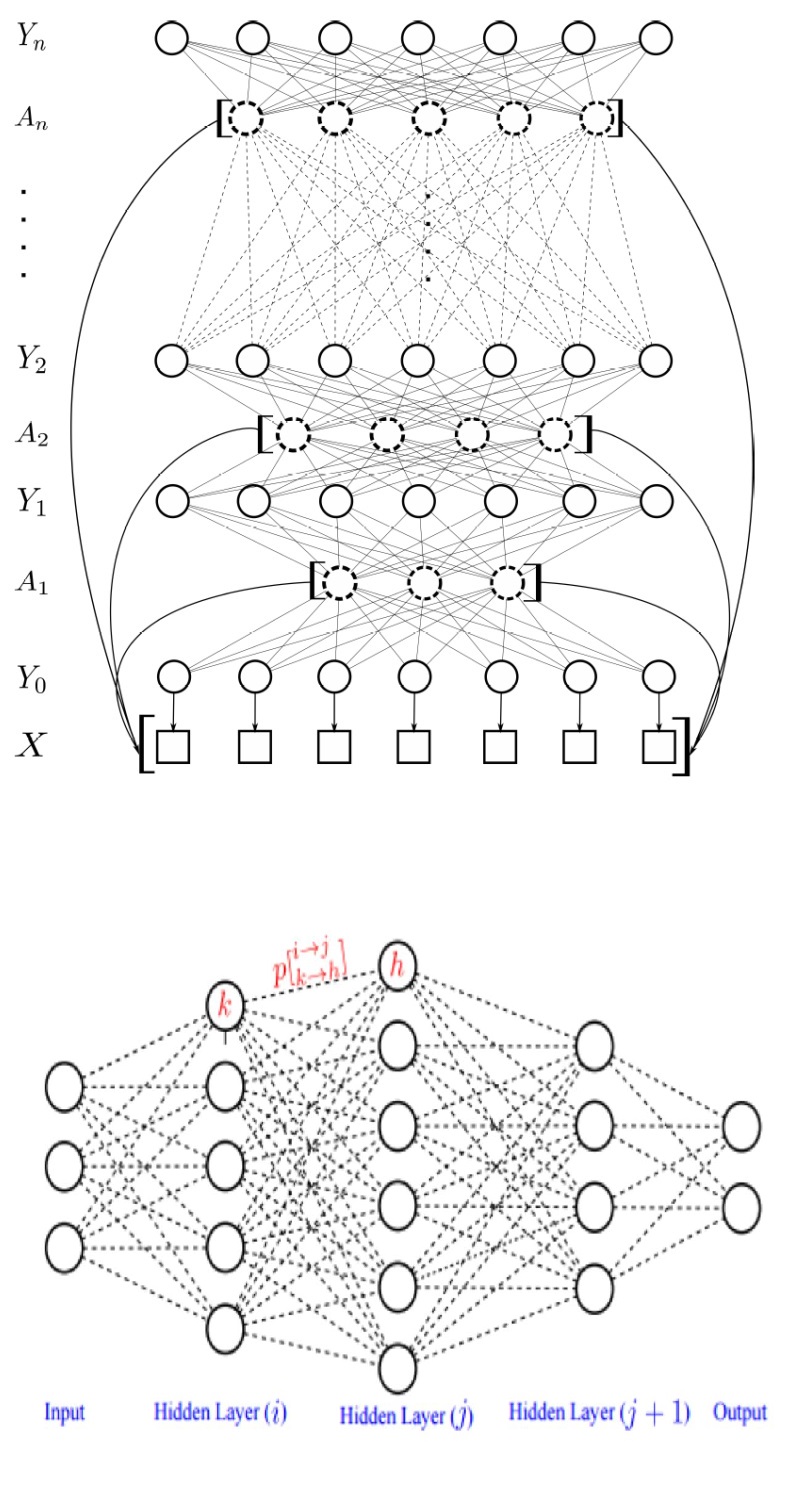
RANDOM DEEP INTELLIGENCE VIA DEEP-STRUCTURED FULLY-CONNECTED GRAPHICAL MODELS
Structured inference, where the goal is to infer a structured state output from a structured observation input, is a crucial component for a wide range of applications such as object recognition, image classification, natural language processing, gesture recognition, handwriting recognition, and bioinformatics. There has been tremendous interest by the scientific community in the use of fully-connected graphical models and deep-structured graphical models for the purpose of structured inference. However, fully-connected and deep-structured graphical models have been largely explored independently, leaving the unification of these two concepts ripe for exploration. A fundamental challenge with unifying these two types of graphical models is in dealing with computational complexity.
My team at Waterloo have developed several pioneering strategies for unifying fully-connected and deep-structured graphical models in such a way that it can be utilized for accurate structured inference in a highly efficient manner, resulting in the notion of random deep intelligence. The innovative deep-structured fully-connected graphical models we developed have been harnessed for forming neural networks and random fields that are able to provide state-of-the-art structured inference performance that are superior to existing models for different applications such as image understanding, while maintaining a high level of computational efficiency.
For more information on the efficient deep-structured fully-connected graphical models we have developed, please read:
1. M. Shafiee, P. Siva, P. Fieguth, and A. Wong, Real-Time Embedded Motion Detection via Neural Response Mixture Modeling. Journal of Signal Processing Systems, 2017.
2. M. Shafiee, P. Siva, and A. Wong, StochasticNet: Forming Deep Neural Networks via Stochastic Connectivity. IEEE Access, 2016.
3. M. Shafiee, P. Siva, P. Fieguth, and A. Wong, Embedded Motion Detection via Neural Response Mixture Background Modeling. IEEE Computer Vision and Pattern Recognition Workshops, 2016.
4. M. Shafiee, P. Siva, P. Fieguth, and A. Wong, Efficient Feature Learning and Extraction via StochasticNets. IEEE Computer Vision and Pattern Recognition Workshops, 2016.
5. A. Chung, M. Shafiee, and A. Wong, Image Restoration via Deep-Structured Stochastically Fully-Connected Conditional Random Fields (DSFCRFs) for Very Low-Light Conditions. Conference on Computer and Robotic Vision, 2016.
6. A. Wong, M. Shafiee, P. Siva, and X. Wang, A deep-structured fully-connected random field model for structured inference. IEEE Access Journal, 2015.
7. M. Shafiee, P. Siva, P. Fieguth, and A. Wong, Domain Adaptation and Transfer Learning in StochasticNets. Journal of Comptuational Vision and Imaging Systems, 2015.
8. M. Shafiee, A. Chung, D. Kumar, F. Khalvati, M. Haider, and A. Wong, Discovery Radiomics via StochasticNet Sequencers for Cancer Detection. NIPS Workshop on Machine Learning for Healthcare, 2015.
Structured inference, where the goal is to infer a structured state output from a structured observation input, is a crucial component for a wide range of applications such as object recognition, image classification, natural language processing, gesture recognition, handwriting recognition, and bioinformatics. There has been tremendous interest by the scientific community in the use of fully-connected graphical models and deep-structured graphical models for the purpose of structured inference. However, fully-connected and deep-structured graphical models have been largely explored independently, leaving the unification of these two concepts ripe for exploration. A fundamental challenge with unifying these two types of graphical models is in dealing with computational complexity.
My team at Waterloo have developed several pioneering strategies for unifying fully-connected and deep-structured graphical models in such a way that it can be utilized for accurate structured inference in a highly efficient manner, resulting in the notion of random deep intelligence. The innovative deep-structured fully-connected graphical models we developed have been harnessed for forming neural networks and random fields that are able to provide state-of-the-art structured inference performance that are superior to existing models for different applications such as image understanding, while maintaining a high level of computational efficiency.
For more information on the efficient deep-structured fully-connected graphical models we have developed, please read:
1. M. Shafiee, P. Siva, P. Fieguth, and A. Wong, Real-Time Embedded Motion Detection via Neural Response Mixture Modeling. Journal of Signal Processing Systems, 2017.
2. M. Shafiee, P. Siva, and A. Wong, StochasticNet: Forming Deep Neural Networks via Stochastic Connectivity. IEEE Access, 2016.
3. M. Shafiee, P. Siva, P. Fieguth, and A. Wong, Embedded Motion Detection via Neural Response Mixture Background Modeling. IEEE Computer Vision and Pattern Recognition Workshops, 2016.
4. M. Shafiee, P. Siva, P. Fieguth, and A. Wong, Efficient Feature Learning and Extraction via StochasticNets. IEEE Computer Vision and Pattern Recognition Workshops, 2016.
5. A. Chung, M. Shafiee, and A. Wong, Image Restoration via Deep-Structured Stochastically Fully-Connected Conditional Random Fields (DSFCRFs) for Very Low-Light Conditions. Conference on Computer and Robotic Vision, 2016.
6. A. Wong, M. Shafiee, P. Siva, and X. Wang, A deep-structured fully-connected random field model for structured inference. IEEE Access Journal, 2015.
7. M. Shafiee, P. Siva, P. Fieguth, and A. Wong, Domain Adaptation and Transfer Learning in StochasticNets. Journal of Comptuational Vision and Imaging Systems, 2015.
8. M. Shafiee, A. Chung, D. Kumar, F. Khalvati, M. Haider, and A. Wong, Discovery Radiomics via StochasticNet Sequencers for Cancer Detection. NIPS Workshop on Machine Learning for Healthcare, 2015.