Data assimilation

Changes of sea ice conditions are leading to increased shipping and offshore activities in the Arctic. However, these activities are affected by the presence of sea ice, which may be hazardous due to ice drift, ridging and pressure. Changing sea ice conditions, and difficulty predicting these changes, poses risks for humans and activities in the Arctic, in addition to economic feasilibity of an operation.
Predictions of an environmental state are often carried out by optimally combining observations with a model state, taking into account the uncertainties in each. Data assimilation is the method used world-wide to generate optimal initial conditions for weather forecasts.
I have been working on the development of methods to assimilate data from various sensors (passive microwave, active microwave and visual infrared) in a sea-ice forecasting system under development at Environment Canada. While passive microwave sensors have the advantage of being relatively insensitive to cloud cover, the data from these sensors has a coarse spatial resolution. This can lead to blurring of the ice edge and small scale features in the ice cover. Data from visual/infrared sensors is higher resolution, but the surface is not visible during cloudy conditions. I have done some work on combining these two data types in the data assimilation system. This is an active research area as the sea ice extent in the Arctic is changing rapidly . This is leading to increased ship activity in ice-infested regions, and hence increased demands on ice services to provide timely information regarding ice conditions.
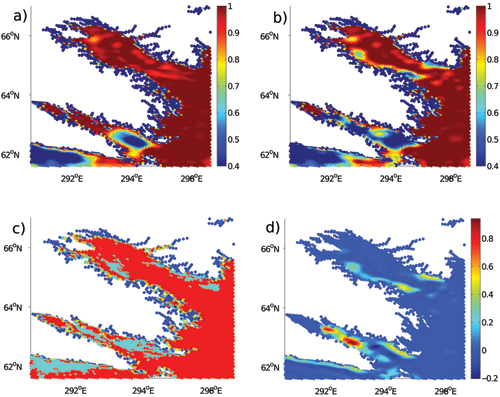
While the assimilation of information pertaining to sea ice concentration is becoming a common part of most operational data assimilation systems, the assimilation of sea ice thickness information is still a relatively unexplored area. Sea ice thickness, particularly that of thin ice, has a critical impact on the heat transferred between the ocean and the atmosphere, and hence on weather near ice-covered regions. Sea ice thickness can be derived from passive microwave, visual infrared or active microwave data, although each of these sources has limitations. A new sensor, Cryosat-2 is providing more direct estimates of sea ice thickness. I have been working on estimating the accuracy of these data sources, in preparation for assimilation experiments.
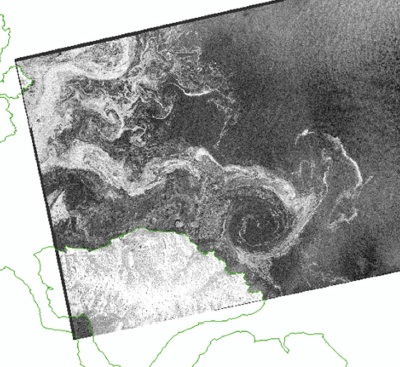
Selected Publications
Jounal papers
C. Cooke and K.A. Scott (2018), Estimating Sea Ice Concentration: Training Convolutional Neural Networks with passive microwave data, accepted for publication in IEEE Transactions on Geoscience and Remote Sensing, doi:10.1109/TGRS.2019.2892723 Available online
N. Asadi, K.A. Scott and D.A. Clausi (2019), Assimilation of ice thickness observations using a regularization framework, accepted for publication in Tellus A, ZELA-2018-0001. Available online
J. Liu, K.A. Scott and P. Fieguth (2018) Detection of Marginal Ice Zone in SAR Imagery using Curvelet based Features: A case study on the Canadian East Coast, accepted for publication in the Journal of Applied Remote Sensing, JARS 210507
K.A. Scott, C. Chen, P.G. Myers, (2018) Assimilation of Argo temperature and salinity profiles using a bias-aware EnOI scheme for the Labrador Sea, accepted for publication in the Journal of Atmospheric and Oceanic Technology, JTECH-S-17-00274.
G. Stonebridge, K.A. Scott and M. Buehner, (2018) The impact of assuming a diagonal obervation error covariance matrix on sea ice analyses: Experiments using a 1D toy model, Tellus A, 70, doi.org/10.1080/16000870.2018.1445379. Available online
L. Wang, K.A. Scott and D. Clausi, (2017), Sea ice concentration estimation during freeze-up from SAR imagery using a convolutional neural network, Remote Sensing, Special issue on Learning to Understand Remote Sensing Images, 9(5), doi:10.3390/rs9050408. Available online
L. Pogson, T. Geldsetzer, M. Buehner, T. Carrieres, M. Ross and K. Andrea Scott, (2017) Collecting empirically-derived SAR characteristic values over one year of sea ice environments for use in data assimilation, Monthly Weather Review, 145, 323-334.
H. Kheyrollah Pour, C.R. Duguay, K.A. Scott and K. Kang, (2017) Improvement of lake ice thickness retrieval from MODIS satellite data using a theromodynamic model, IEEE Transactions on Geoscience and Remote Sensing, 55(10), doi:10.1109/TGRS.2017.2718533.
J. Liu, K.A. Scott, A. Gawish and P. Fieguth, (2016) Automatic detection of the ice edge in SAR imagery using curvelet transform and active contour, Remote Sensing, 8(6), doi:10.3390/rs8060480. Available online
L. Wang, K.A. Scott and D. Clausi, (2016) Improved sea ice concentration estimation through fusing classified SAR imagery and AMSR-E data, Canadian Journal of Remote Sensing, 42(1), doi:10.1080/07038992.2016.1152547 Available online
L. Wang, K.A. Scott, L. Xu and D.A. Clausi, (2016) Sea ice concentration estimation during melt from dual-pol SAR scenes using deep convolutional neural networks: A case study, IEEE Transactions on Geoscience and Remote Sensing, 54(8), 4524-4533, doi:10.1109/TGRS.2016.2543660. Available online
K.A. Scott, Z. Ashouri, M. Buehner, L. Pogson and T. Carrieres, (2015) Assimilation of ice and water observations from SAR imagery to improve estimates of sea ice concentration, Tellus A, 67, 27218, http://dx.doi.org/10.3402/tellusa.v67.27218 Available online
W. Tan, K.A. Scott and E. LeDrew (2014) Enhanced Arctic sea ice concentration merging MODIS sea ice surface temperature and SSM/I sea ice concentration, Atmosphere-Ocean, 52(2), 115-124.
K.A. Scott, A. Wong and E. Li (2014) Sea ice surface temperature estimation using MODIS and AMSR-E data within a guided variational model along the Labrador Coast, IEEE Journal of Selected Topics in Applied Earth Observations and Remote Sensing, 7(9), 3685-3694.
K.A. Scott, M. Buehner and T. Carrieres (2014) An assessment of sea ice thickness from AMSR-E and MODIS data for operational data assimilation, IEEE Transactions on Geoscience and Remote Sensing, 52(5), 2726-2738.
K.A. Scott, M. Buehner, A. Caya and T. Carrieres, (2012) A preliminary evaluation of the impact of assimilating AVHRR data on sea ice concentration analyses, Remote Sensing of Environment, Vol. 128, p.212-223
K.A. Scott, M. Buehner. A. Caya and T. Carrieres (2012) Direct assimilation of AMSR-E brightness temperatures to estimate sea ice concentration, Monthly Weather Review, Vol. 140, p.997-1013
Conference papers
Y. Xu and K.A. Scott (2017) Sea ice and open water classification of SAR imagery using CNN-based transfer learning, accepted for the IEEE International Geoscience and Remote Sensing Symposium, Fort Worth, USA, 2017, 4p.
J. Liu, K.A. Scott and P. Fieguth (2015) Curvelet based feature extraction of dynamic ice from SAR imagery, accepted for IEEE International Geoscience and Remote Sensing Symposium, Milan, Spain, 2015. 4p.
Z. Ashouri, K.A. Scott and T. Carrieres (2013) Effect of Denoising on Assimilation of SAR data, presented at SPIE Remote Sensing of the Ocean, Sea Ice, Coastal Water and Large Water Regions, Dresden Germany, September 23-26, 2013, 7p.
A. Wong, K.A. Scott, E. Li and R. Amelard (2013) Continuous sea ice thickness estimation using a joint MODIS and AMSR-E guided variation model, accepted for IEEE International Geoscience and Remote Sensing Symposium, Melborne Australia, 2013. 4p.
K.A. Scott, Z. Ashouri, M. Buehner, L. Pogoson and T. Carrieres (2013) Assimilation of SAR data in the marginal ice zone, accepted for IEEE Radar Conference, Ottawa, Canada, 2013. 6p.